New Acoustic Attack – Deep Learning Model Extracts Keystrokes with 95% Accuracy
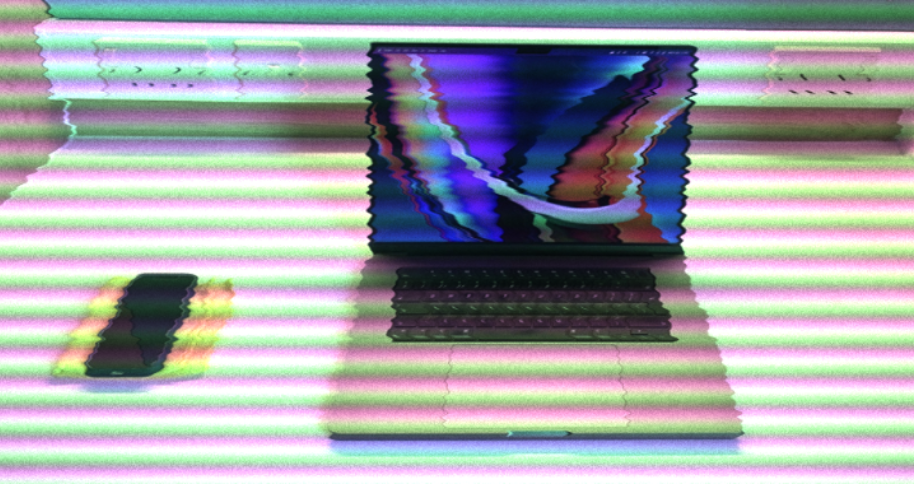
A team of researchers hailing from British universities has unveiled a disconcerting breakthrough in the realm of cyber threats — a deep learning model that can pilfer sensitive data by capturing keyboard keystrokes using a microphone, boasting a remarkable accuracy of 95%.
The researchers found that even when the sound classification algorithm was trained using Zoom recordings, the prediction accuracy only slightly dipped to 93%, still posing a grave risk. Such an attack poses a severe threat to data security, as it has the potential to expose passwords, confidential discussions, messages, and other sensitive information to malicious actors.
What sets this method apart from other side-channel attacks is its simplicity and versatility. Unlike other attacks that necessitate specific conditions and are limited by data rates and distances, acoustic attacks have become notably uncomplicated due to the ubiquity of devices equipped with microphones capable of capturing high-quality audio.
See Also: So you want to be a hacker?
Offensive Security, Bug Bounty Courses
This heightened ease of execution, coupled with rapid advancements in machine learning, magnifies the potency of sound-based side-channel attacks, rendering them more perilous than previously anticipated.
Keystrokes Listening
The attack process involves capturing keyboard keystrokes, which serve as the training data for the prediction algorithm. This data can be acquired via a nearby microphone or even a compromised smartphone with microphone access due to malware infiltration. Additionally, a rogue participant in a Zoom call can exploit correlations between typed messages and recorded sounds to achieve the same outcome.
To gather training data, the researchers meticulously pressed 36 keys on a modern MacBook Pro, recording the sound generated by each keystroke.
Sampling the keystroke audio (arxiv.org)
The resulting waveforms and spectrograms exhibited distinct patterns for each key, which were then processed to enhance signals for keystroke identification.
Produced spectrograms (arxiv.org)
The researchers employed the spectrogram images to train an image classifier named ‘CoAtNet.’ Through iterations of experimentation with parameters like epoch, learning rate, and data splitting, the algorithm’s prediction accuracy was optimized.
Parameters selected for training CoAtNet (arxiv.org)
Tests were conducted using a standard laptop keyboard, an iPhone 13 mini positioned 17cm away from the target, and Zoom.
The test setup (arxiv.org)
Impressively, the CoAtNet classifier achieved a 95% accuracy rate from smartphone recordings and a 93% accuracy rate from Zoom-captured data. Even the lower but still significant 91.7% accuracy was attained using Skype.
Confusion matrix for phone-recorded keystrokes (arxiv.org)
Trending: Recon Tool: RecoX
Mitigation Strategies
In light of these revelations, the researchers offer some possible mitigation strategies. For individuals concerned about acoustic side-channel attacks, adopting varied typing styles or utilizing randomized passwords may be helpful. Employing software to simulate keystroke sounds, introducing white noise, or implementing software-based keystroke audio filters are potential defense measures.
However, it is important to note that the efficacy of the attack remained intact even with exceptionally silent keyboards, rendering solutions such as sound dampeners for mechanical keyboards or transitioning to membrane-based keyboards ineffective.
Ultimately, employing biometric authentication where feasible and utilizing password managers to eliminate the necessity of manual input for sensitive information also contribute to bolstering defense against these emerging threats.
Are u a security researcher? Or a company that writes articles or write ups about Cyber Security, Offensive Security (related to information security in general) that match with our specific audience and is worth sharing?
If you want to express your idea in an article contact us here for a quote: [email protected]
Source: bleepingcomputer.com